Quantitative OCTA analysis and AI classification of eye diseases
The retina, located at the posterior part of the eye, is responsible for converting light into bioelectrical signals and transmitting them to the central nervous system. As a component of the CNS, the retina is composed of multiple layers of neural and vascular tissue, making it vulnerable to systemic conditions like diabetes and sickle cell anemia. These diseases can lead to diabetic retinopathy (DR) and sickle cell retinopathy (SCR), which often remain asymptomatic until irreversible damage occurs. Early detection and intervention are critical for preventing or slowing down disease progression. To visualize retinal changes and diagnose these diseases, various retinal imaging techniques such as fundus photography, optical coherence tomography (OCT), and OCT angiography (OCTA) are used. Fundus photography is a quick and relatively inexpensive technique, but it has limited resolution to visualize microcapillary changes. OCT provides high-resolution 3D cross-sectional information to visualize individual retinal layers. OCTA provides high-resolution visualization of the retina vasculature. However, current screening methods rely on manual grading by trained graders, leading to grading errors. Thus, developing quantitative features is crucial for reliable diagnosis.
Figure Heading link
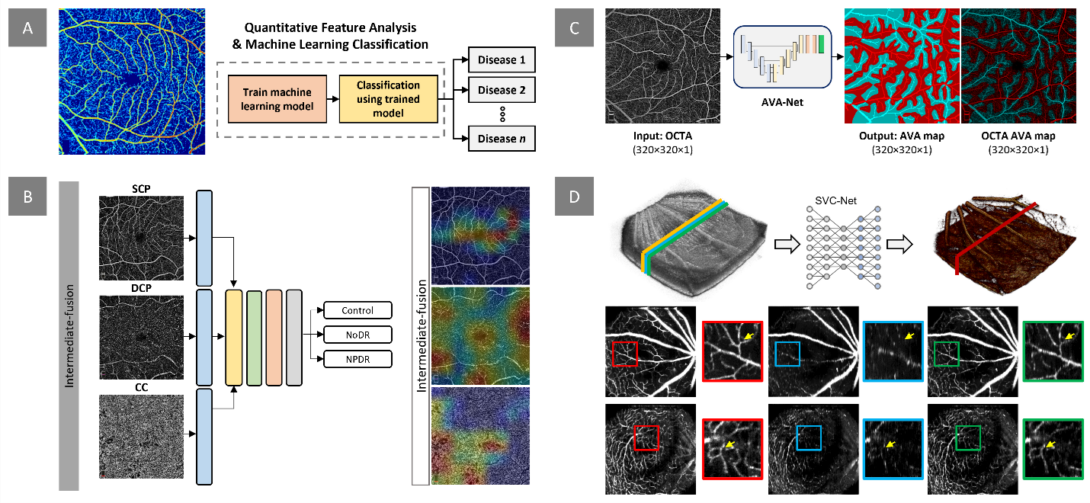
Figure. Our research project on quantitative OCTA analysis and AI classification focuses on several areas. A) We aim to develop digital biomarkers for early disease detection and evaluation of treatment outcomes. This example demonstrates the measurement of normalized blood flow index, which can be used to train machine learning models for disease detection and staging. B) In addition to extracting quantitative features, we design and implement deep learning models for automated classification of retinopathy using OCTA images. Our recent work investigates the effects of multi-layer OCTA fusion for robust diabetic retinopathy classification. C) Clinical observations indicate that retinopathies affect arteries and veins differently. To differentiate between different retinopathy stages, we employ differential artery-vein (AV) analysis. Our recent work explores deep learning-based AV-Area (AVA) segmentation for this purpose. D) Traditional OCTA processing requires repeated scans, leading to increased acquisition time and potential motion artifacts. To address this, we propose a deep learning approach that utilizes the spatial vascular connectivity (SVC) between scans in a single-scan volumetric OCT for OCTA prediction. Overall, our research project combines quantitative OCTA analysis and AI classification techniques to advance the field of retinopathy detection and characterization.
Current development and future direction Heading link
Quantitative retinal feature analysis
Visualization of the retina vasculature. These features include blood vessel caliber, blood vessel tortuosity, foveal avascular zone (FAZ) contour irregularity, and branchpoint angle features. We have also developed differential AV analysis, which involves analyzing the differences between the arterial and venous components of the retinal vasculature, to improve disease staging. For neuronal changes, we derive various features in optical coherence tomography (OCT), which provides high-resolution 3D cross-sectional information to visualize individual retinal layers. One of these features is the intensity of the hyperreflective bands of the outer retina, which can show early changes in DR patients. Additionally, we are exploring other OCT features, such as the thickness of the ganglion cell layer, inner nuclear layer, and outer plexiform layer, as well as the volume of the macular and peripapillary regions, to quantify neuronal changes associated with retinal diseases.
AI Implementation: Machine learning and Deep learning
Using quantitative features, we utilize machine learning algorithms for the automated classification of different retinopathies, including DR and SCR. We also demonstrate that quantitative OCTA features can be used for multi-disease classification and staging. In recent studies, we have explored deep learning data fusion in OCTA and fundus photography to improve classification performance. Our projects also focus on developing deep learning technologies for computer vision tasks, such as image segmentation and reconstruction. Specifically, we have developed several deep learning models, including AV-Net, MF-AV-Net, and AVA-Net, for automated AV segmentation. Additionally, we have demonstrated the effectiveness of ADC-Net for deep learning-based automated dispersion compensation, and we have developed SVC-Net for deep learning-based OCTA construction from single-scan volumetric OCT. The use of deep learning in ophthalmology holds tremendous promise and has the potential to revolutionize the field of computer vision.
Future direction
Our future research directions involve both feature development and machine learning algorithms to improve the accuracy and efficiency of disease detection. We plan to focus on further quantifying specific neurovascular changes using traditional machine learning models and deep learning algorithms. The goal is to develop reliable and robust quantitative features that can be integrated into a computer-aided diagnosis (CAD) system for automated disease diagnosis and staging. By doing so, we can reduce the need for manual inspection by trained graders, minimizing grading errors and enabling earlier intervention for better patient outcomes. Ultimately, our aim is to improve the efficiency and accuracy of disease detection by developing a set of reliable and robust quantitative features.
Related publications Heading link
2024
- Le, D., Son, T., Kim, TH. et al. Deep learning-based optical coherence tomography angiography image construction using spatial vascular connectivity network. Commun Eng 3, 28 (2024). https://doi.org/10.1038/s44172-024-00173-9. [Full text]
2023:
- Ebrahimi, Behrouz, David Le, Mansour Abtahi, Albert K. Dadzie, Jennifer I. Lim, RV Paul Chan, and Xincheng Yao. “Optimizing the OCTA layer fusion option for deep learning classification of diabetic retinopathy.” Biomedical Optics Express 14, no. 9 (2023): 4713-4724. [Full text]
- Le, David, Mansour Abtahi, Tobiloba Adejumo, Behrouz Ebrahimi, Albert K Dadzie, Taeyoon Son, and Xincheng Yao.”Deep learning for artery–vein classification in optical coherence tomography angiography.” Experimental Biology and Medicine. 2023;0(0). doi:10.1177/15353702231181182. [Full text]
- Abtahi, Mansour, David Le, Behrouz Ebrahimi, Albert K. Dadzie, Jennifer I. Lim, and Xincheng Yao. “An open-source deep learning network AVA-Net for arterial-venous area segmentation in optical coherence tomography angiography.” Communications Medicine 3, no. 1 (2023): 54.[Full text]
- Dadzie, Albert K., David Le, Mansour Abtahi, Behrouz Ebrahimi, Taeyoon Son, Jennifer I. Lim, Xincheng Yao.”Normalized Blood Flow Index in Optical Coherence Tomography Angiography Provides a Sensitive Biomarker of Early Diabetic Retinopathy.” Trans. Vis. Sci. Tech. 2023;12(4):3.[Full text]
- Le, David, Taeyoon Son, Tae-Hoon Kim, Tobiloba Adejumo, Mansour Abtahi, Shaiban Ahmed, Alfa Rossi, Behrouz Ebrahimi, Albert Dadzie, and Xincheng Yao. “SVC-Net: A spatially vascular connectivity network for deep learning construction of microcapillary angiography from single-scan-volumetric OCT.” (2023). [Full text]
- Le, David, Albert Dadzie, Taeyoon Son, Jennifer I. Lim, and Xincheng Yao. “Comparative Analysis of OCT and OCT Angiography Characteristics in Early Diabetic Retinopathy.” RETINA (2023): 10-1097. [Full text]
2022:
- Abtahi, Mansour, David Le, Jennifer I. Lim, and Xincheng Yao. “MF-AV-Net: an open-source deep learning network with multimodal fusion options for artery-vein segmentation in OCT angiography.” Biomedical Optics Express 13, no. 9 (2022): 4870-4888. [Full text]
- Ahmed, Shaiban, David Le, Taeyoon Son, Tobiloba Adejumo, Xincheng Yao, and Visual Science. “ADC-Net: An Open-Source Deep Learning Network for Automated Dispersion Compensation in Optical Coherence Tomography.” Frontiers in Medicine (2022). [Full text]
- Le, David, Taeyoon Son, Jennifer I. Lim, and Xincheng Yao. “Quantitative optical coherence tomography reveals rod photoreceptor degeneration in early diabetic retinopathy.” Retina 2022. [Full text]
2021
- Alam, Minhaj Nur, David Le, Taeyoon Son, Jennifer I. Lim, and Xincheng Yao. “Quantitative features for objective assessment of OCT angiography.” In Photo Acoustic and Optical Coherence Tomography Imaging, Volume 3: Angiography: an application in vessel imaging. IOP Publishing, 2021. [Full text]
- Le, David, Taeyoon Son, and Xincheng Yao. “Machine learning in optical coherence tomography angiography.” Experimental Biology and Medicine (2021): 15353702211026581. [Full text]
- Alam, Minhaj Nur, David Le, and Xincheng Yao. Differential artery-vein analysis in quantitative retinal imaging: a review. Quant Imaging Med Surg 2021;11:1102-19 [Full text]
- Alam, Minhaj Nur, David Le, Jennifer I. Lim, and Xincheng Yao. Vascular Complexity Analysis in Optical Coherence Tomography Angiography of Diabetic Retinopathy. Retina 2021;41:538-45 [Full text]
2020
- Alam, Minhaj, David Le, Taeyoon Son, Jennifer I. Lim, and Xincheng Yao, “AV-Net: deep learning for fully automated artery-vein classification in optical coherence tomography angiography,” Biomed. Opt. Express 11, 5249-5257 (2020) [Full text]
- Le, David, Minhaj Alam, Cham K. Yao, Jennifer I. Lim, Yi-Ting Hsieh, Robison VP Chan, Devrim Toslak, and Xincheng Yao. “Transfer learning for automated OCTA detection of diabetic retinopathy.” Translational Vision Science & Technology 9, no. 2 (2020): 35-35. [Full text]
- Alam, Minhaj Nur, Yue Zhang, Jennifer I. Lim, Robison VP Chan, Min Yang, and Xincheng Yao. “Quantitative optical coherence tomography angiography features for objective classification and staging of diabetic retinopathy.” Retina 40, no. 2 (2020): 322-332. [Full Text].
2019
- Alam, Minhaj, David Le, Jennifer I. Lim, R.V.P. Chan, Xincheng Yao, “Supervised machine learning based multi-task artificial intelligence classification of retinopathies,” Journal of Clinical Medicine (2019) [Full text].
- Hsieh, Yi-Ting, Minhaj Nur Alam, David Le, Chia-Chieh Hsiao, Chang-Hao Yang, Daniel L. Chao, Xincheng Yao. “Optical Coherence Tomography Angiography Biomarkers for Predicting Visual Outcomes after Ranibizumab Treatment for Diabetic Macular Edema.” Ophthalmology Retina , Volume 3, Issue 10, 826 – 834 (2019) [Full text]
- Le, David, Minhaj Alam, Bernadette A. Miao, Jennifer I. Lim, and Xincheng Yao. “Fully automated geometric feature analysis in optical coherence tomography angiography for objective classification of diabetic retinopathy.” Biomedical optics express 10, no. 5 (2019): 2493-2503. [Full text]
- Alam, Minhaj, Devrim Toslak, Jennifer I. Lim, and Xincheng Yao. “OCT feature analysis guided artery-vein differentiation in OCTA.” Biomedical optics express 10, no. 4 (2019): 2055-2066. [Full Text]
- Alam, Minhaj, Jennifer I. Lim, Devrim Toslak, and Xincheng Yao. “Differential artery–vein analysis improves the performance of OCTA staging of sickle cell retinopathy.” Translational vision science & technology 8, no. 2 (2019): 3-3. [Full Text]
2018
- Alam, Minhaj, Devrim Toslak, Jennifer I. Lim, and Xincheng Yao. “Color fundus image guided artery-vein differentiation in optical coherence tomography angiography.” Investigative ophthalmology & visual science 59, no. 12 (2018): 4953-4962. [Full Text]
- Alam, Minhaj, Taeyoon Son, Devrim Toslak, Jennifer I. Lim, and Xincheng Yao, “Combining optical density ratio and blood vessel tracking for automated artery-vein classification and quantitative analysis in color fundus images”. Translational Vision Science & Technology (TVST) 7(2): 23 (2018) [Full Text]
2017
- Alam, Minhaj, Damber Thapa, Jennifer I. Lim, Dingcai Cao, and Xincheng Yao. “Computer-aided classification of sickle cell retinopathy using quantitative features in optical coherence tomography angiography.” Biomedical optics express 8, no. 9 (2017): 4206-4216.[Full Text]
- Alam, Minhaj, Damber Thapa, Jennifer I. Lim, Dingcai Cao, and Xincheng Yao. “Quantitative characteristics of sickle cell retinopathy in optical coherence tomography angiography.” Biomedical optics express 8, no. 3 (2017): 1741-1753. [Full Text]